Publications
Contrastive Sparse Autoencoders for Interpreting Planning of Chess-Playing Agents
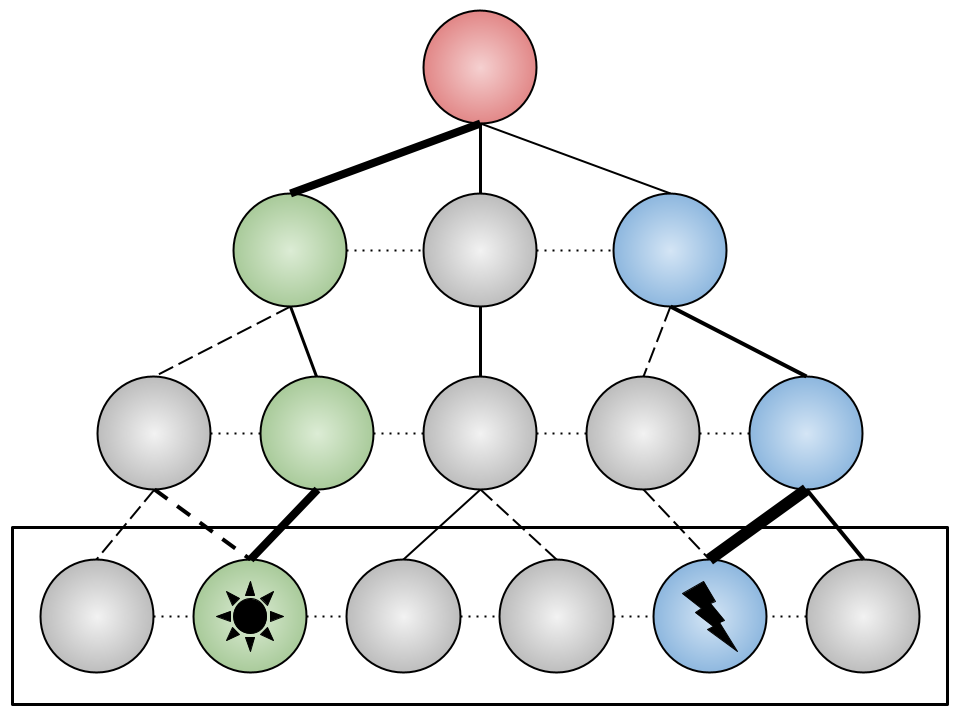
We propose contrastive sparse autoencoders (CSAE), a novel framework for studying pairs of game trajectories. Using CSAE, we are able to extract and interpret concepts that are meaningful to the chess-agent plans. We primarily focused on a qualitative analysis of the CSAE features before proposing an automated feature taxonomy. Furthermore, to evaluate the quality of our trained CSAE, we devise sanity checks to wave spurious correlations in our results.
Perspectives for Direct Interpretability in Multi-Agent Deep Reinforcement Learning
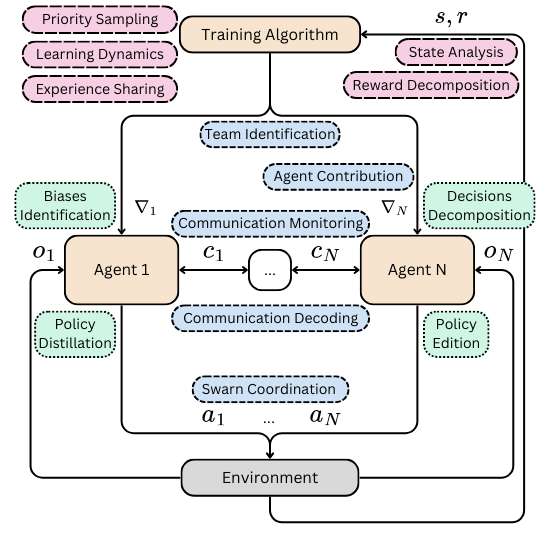
By addressing Multi-Agent Deep Reinforcement Learning interpretability, we propose directions aiming to advance active topics such as team identification, swarm coordination and sample efficiency. This paper advocates for direct interpretability, generating post hoc explanations directly from trained models, offering scalable insights into agents' behaviour, emergent phenomena, and biases without altering models' architectures.